
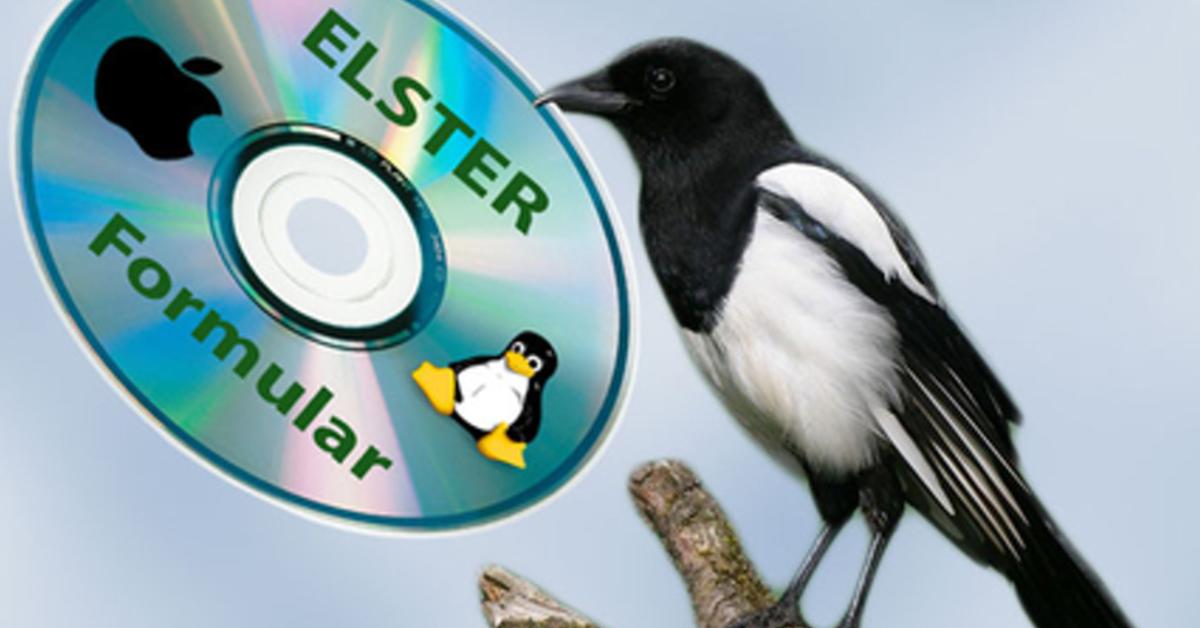
The house has the original wood-frame construction. This results in an inability to reproduce and compare algorithms published. This means that the same dataset can be cleaned very differently. Other datasets (e.g., REDD 14) leave the onus of data cleaning on each researcher. For electricity data we provide 11 measurement characteristics for each meter: voltage, current, frequency, displacement power factor, apparent power factor, real power, real energy, reactive power, reactive energy, apparent power, and apparent energy.ĪMPds2 data has been cleaned to provide for consistent and comparable accuracy results amongst different researchers and machine learning algorithms. Data is captured over a long period of time (two years) and is presented with minor amounts of missing data algorithmically filled in to maintain a continuous frequency of readings (once per minute). It is the only dataset to include all three main types of consumption: electricity, water, and natural gas. However, AMPds2 is unique for a number of different reasons. There are indeed other datasets that exist from the USA 12– 16, Europe 17– 21, and Asia 22. Synthesized data does not realistically represent an actual dataset as ‘a real-world dataset would normally have certain complexity that is harder predict and in many cases can be very difficult to deal with’. Testing the accuracy performance with real-world datasets is crucial in these fields of research.
ELSTER ONLINE FORMULAR 2014 VERIFICATION
load disaggregation) 8, energy use behaviour, eco-feedback and eco-visualizations 6, 9, application and verification of theoretical algorithms/models, appliance studies, demand forecasting, smart home frameworks, grid distribution analysis, time-series data analysis, energy efficiency studies, occupancy detection, energy polity and socio-economic frameworks 10, and advanced metering infrastructure (AMI) analytics. We have added historical climate data, two years of hourly weather data, and two years of utility billing data.ĪMPds has been used (See Google Scholar: ) and can be used in research that looks at: non-intrusive load monitoring (NILM, a.k.a. The integrity problems that existed in the first release have been corrected. With this second release (AMPds2) we increased the monitoring length to two years (730 days of captured data per meter). The first release also contained some data integrity issues: missing readings and a counter reset that happened with the water meters. The Almanac of Minutely Power dataset (AMPds) was initially released in 2013 with one year of meter data without environmental and utility billing data 7 ( Data Citation 1). One way to help homeowners and occupants reduce their consumption is to monitor and present how much power their appliances are using through an effective eco-feedback device or display mechanism 5, 6. Residential homes contribute about 34% to the total power consumption in the USA and their consumption is projected to increase to 39% by 2030 (ref. As the cost of power rises, we must find technological solutions that help reduce and optimize energy use 2, 3. Additionally, there is a growing consensus that environmental and economical sustainability are inextricably linked 1. AMPds2 data has been pre-cleaned to provide for consistent and comparable accuracy results amongst different researchers and machine learning algorithms.Ĭurrently, much of the world is focused on reducing electricity consumption our increase in consumption is neither economically nor environmentally sustainable. We also include environmental and utility billing data for cost analysis. Each meter has 730 days of captured data. No other such datasets from Canada exist. AMPds2 is the first dataset to capture all three main types of consumption (electricity, water, and natural gas) over a long period of time (2 years) and provide 11 measurement characteristics for electricity. In the vast majority of cases, real-world datasets lead to more accurate models and algorithms. The Almanac of Minutely Power dataset Version 2 (AMPds2) has been released to help computational sustainability researchers, power and energy engineers, building scientists and technologists, utility companies, and eco-feedback researchers test their models, systems, algorithms, or prototypes on real house data. With the cost of consuming resources increasing (both economically and ecologically), homeowners need to find ways to curb consumption.
